Medical image analysis encompasses two crucial research areas: disease grading and fine-grained lesion segmentation. Although disease grading often relies on fine-grained lesion segmentation, they are usually studied separately. Disease severity grading can be approached as a classification problem, utilizing image-level annotations to determine the severity of a medical condition. On the other hand, fine-grained lesion segmentation requires more detailed pixel-level annotations to precisely outline and identify specific abnormalities in the images. However, obtaining pixel-wise annotations of medical images is a time-consuming and resource-intensive process as it requires domain experts. Moreover, deep learning-based disease diagnosis models often perform poorly in real-life scenarios due to a lack of explainability and domain discrepancies.
In this work, we leverage interactive machine learning to introduce a joint learning framework, DRG-Net [1], for automatic multi-lesion identification and disease grading for Diabetic Retinopathy. It is an ocular disease that damages the retina’s blood vessels, and it is one of the leading causes of irreversible blindness. DRG-Net includes a novel Domain Invariant Lesion Feature Generator and Attention-Based Disease Grading Classifier. The former aims to identify and distinguish important lesion regions on a retinal fundus/OCT image, while the latter incorporates the predicted lesion information in the learning process of the disease classification network. We formulate a novel domain adaptation constraint to adversarially train DRG-Net under labeled data scarcity. Our framework is designed as a collaborative learning system between users and the machine learning model, allowing users to inspect predicted results and provide feedback on the model’s outputs to interactively fine-tune the model for enhanced performance.
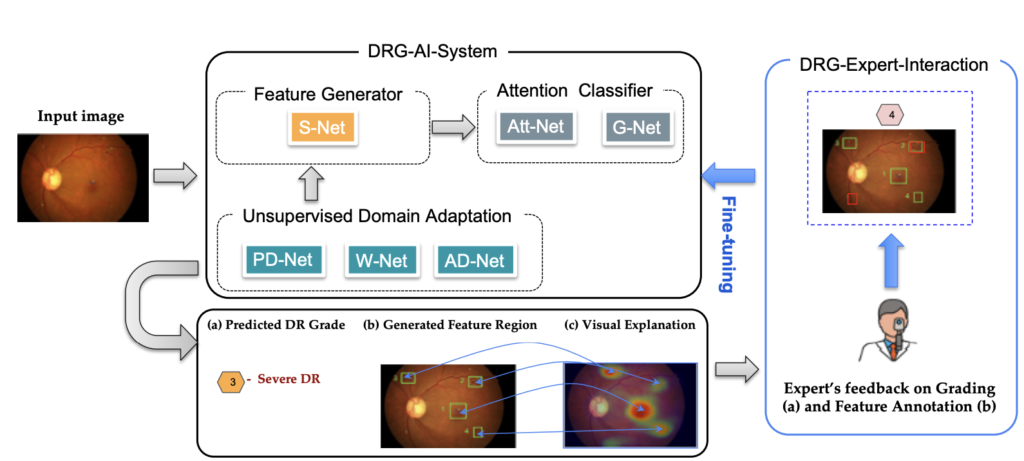
Figure 1: A high-level overview of our DRG-Net system that contains two main modules, DRG-AI-System: Given a retinal image, our deep learning models will simultaneously generate three outcomes prediction (DR grade, localized lesion regions, and visual explanation). It consists of networks for lesion feature generators and attention-based classifiers, which are simultaneously optimized under unsupervised domain adaptation constraints. DRG-Expert-Interaction: By using an Intelligent User Interface (IUI), ophthalmologists can observe the system’s predictions and provide feedback to fine-tune the model through an active learning cycle.
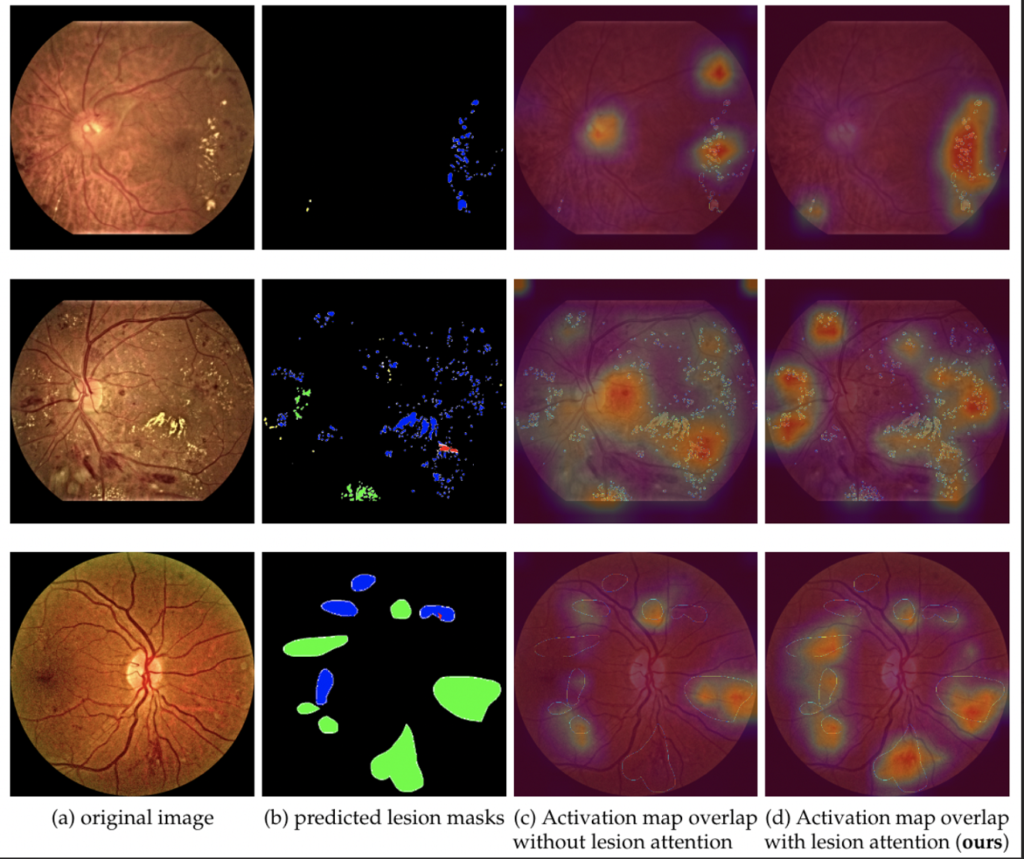
Figure 2: Comparison of explainability of the disease classification model trained with and without lesion attention. (a) original inputs, (b) union of ground-truth lesion maps, (c) & (d) depicts the overlapping of class activation map (CAM) of the predicted grading class with the (a) and (b) for classification trained with/without lesion attention respectively. We can observe that CAMs in column (d) for DRG-Net overlap with most of the lesion regions.
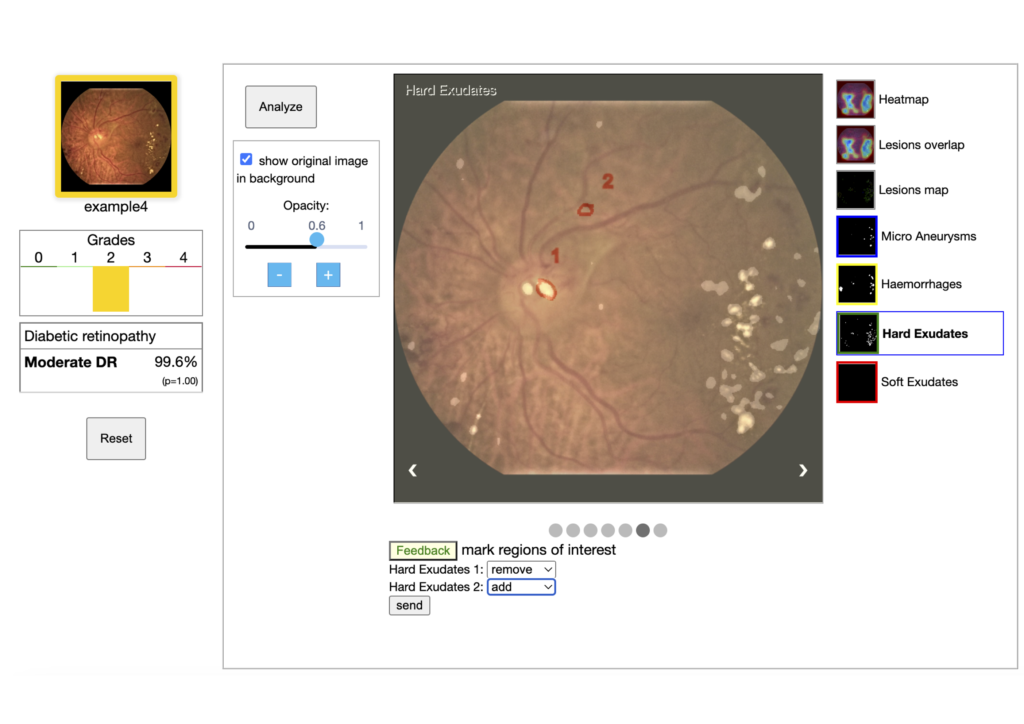
Figure 3: The user interface of the DRG-Net demo. For a given input image, the AI models provide multiple visual information along with disease severity grade. Visual explanations are provided by highlighting associated lesion regions and their overlap on the saliency map of the grading model. User feedback on the addition or deletion of lesion regions is collected using mouse input.
References
[1] Tusfiqur, H. M., Nguyen, D. M., Truong, M. T., Nguyen, T. A., Nguyen, B. T., Barz, M., … & Sonntag, D. (2022). DRG-Net: Interactive Joint Learning of Multi-lesion Segmentation and Classification for Diabetic Retinopathy Grading. arXiv preprint arXiv:2212.14615.