Contract cheating, an academic dishonesty when students ask another person to take an exam or to do an assignment instead of them, turns out to be a significant and growing problem, receiving a large amount of media coverage. It becomes a mature industry with a lot of specialized websites full of ads with offers to sit exams on behalf of students. Proctoring solutions as a countermeasure extensively use video monitoring that is not only prone to false alarms, imposing additional stress on students, but also doubtful in terms of students’ privacy and personal rights.
With this work, we present a new system to prevent contract cheating during exams based on on-line handwriting analysis. The handwriting appearance can be quite expressive. Stroke features have been successfully used to predict domain expertise and cognitive performance. We expect that handwriting style can capture the author individuality, therefore, we propose to use handwriting verification technique for continuous user authentication. To record user’s input we use a digital ball pen: It resembles traditional pen and allows writing on plain paper but is equipped with an optical sensor for digitizing hand-drawn sketches. To capture the uniqueness of the handwriting, we resort to a neural network architecture that is capable of disentangling style from content. Given two samples of handwriting, we use their style encodings to calculate the dissimilarity score between them and decide if they belong to the same writer. Our model is able to score user’s input automatically in real-time.
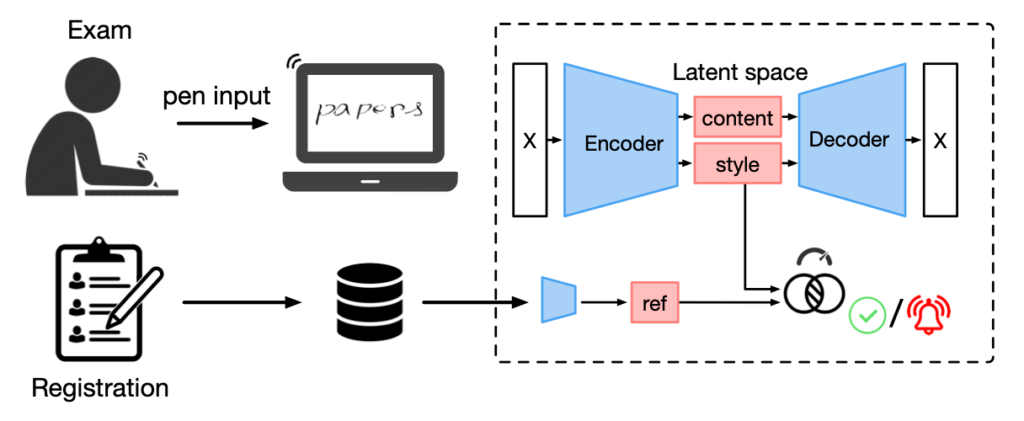
Figure 1
A regular examination is conducted as follows (see Figure 1): Well in advance of the exam students, whose identity can be validated with many factors, fill out a registration form with a digital pen. The handwriting sample is stored in a database and later used as a reference sample. During the exams, the students solve assignments with a digital pen in the same way they would do so with a usual pen and paper. Meanwhile, short samples of their writing are continuously transferred to the server and compared to the reference, thus performing continuous passive authentication. If the system detects that the handwriting does not match the reference, this should be considered a hint for the examiner to check the student’s identity. The system is suitable for both on-site and online exams and can also identify if an impersonator is substituted during an exam.
An evaluation based on the large Deepwriting dataset showed that our system can successfully differentiate between the handwriting styles of different authors and hence detect simulated cases of contract cheating. We also conducted a 7-person validation study using digital ballpoint pens to assess the system’s reliability in a more realistic environment.