Digital pen signals were shown to be predictive for cognitive states, cognitive load and emotion in educational settings. We investigate whether low-level pen-based features can predict the difficulty of tasks in a cognitive test and the learner’s performance in these tasks, which is inherently related to cognitive load, without a semantic content analysis. We record data for tasks of varying difficulty in a controlled study with children from elementary school. We include two versions of the Trail Making Test (TMT) and six drawing patterns from the Snijders-Oomen Non-verbal intelligence test (SON) as tasks that feature increasing levels of difficulty. We examine how accurately we can predict the task difficulty and the user performance as a measure for cognitive load using support vector machines and gradient boosted decision trees with different feature selection strategies. The results show that our correlation-based feature selection is beneficial for model training, in particular when samples from TMT and SON are concatenated for joint modelling of difficulty and time. Our findings open up opportunities for technology-enhanced adaptive learning.
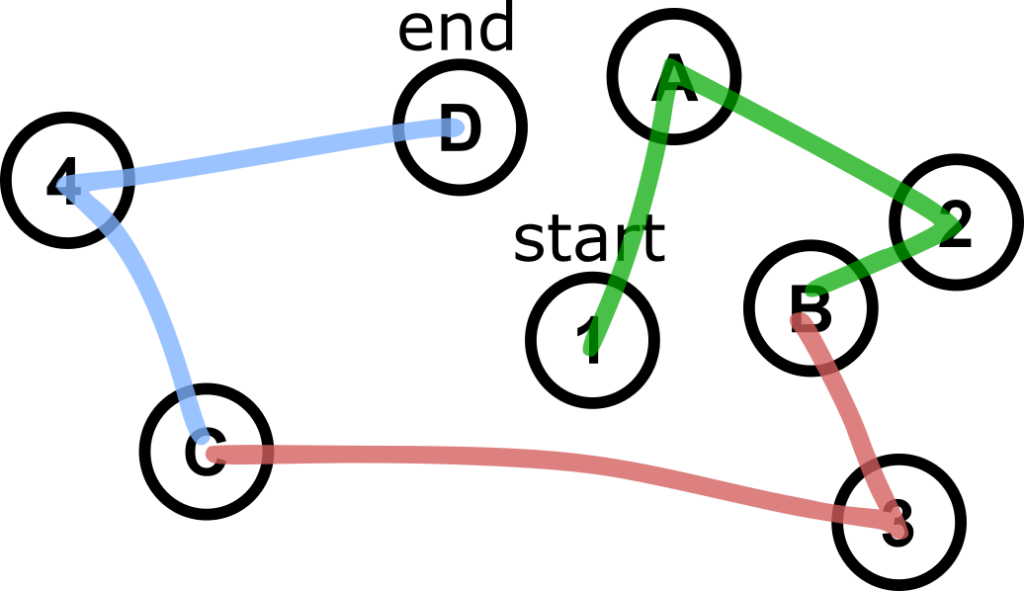
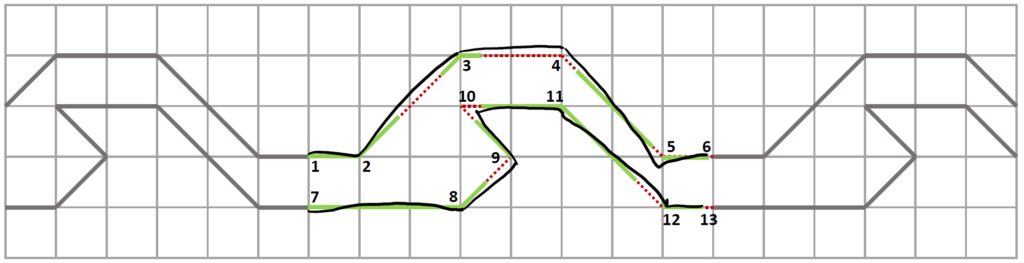
References
- Digital Pen Features Predict Task Difficulty and User Performance of Cognitive Tests. In: Proceedings of the 28th ACM Conference on User Modeling, Adaptation and Personalization, ACM, 2020.