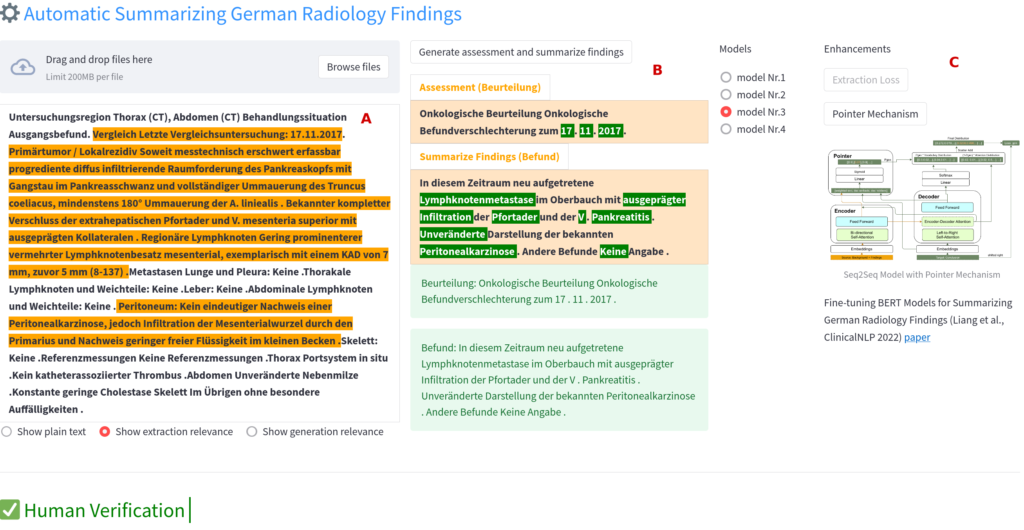
Figure 1: The interface guides annotators in human evaluation. Snippet A displays the original oncology report with highlighted sentences extracted automatically. Snippet C lets annotators choose the model for generation, showing its architecture. The middle section (B) presents the chosen model’s generation results, with green words denoting the most learned and relevant features incorporated into the output.
Writing concise radiology reports to communicate findings is crucial. Weber et al. (2020) introduced Structured Oncology Reporting (SOR) to address traditional reporting challenges. Our focus is on using BERT2BERT-based models to summarize German radiology findings in structured reports. We propose BERT2BERT+Ext and BERT2BERT+Ptr to enhance factual correctness in generated conclusions. Both models show significant improvements with minimal changes to the baseline. BERT2BERT+Ext encourages key sentence reconstruction during training, while BERT2BERT+Ptr uses the pointer mechanism to directly copy relevant segments. Despite limitations and imbalanced data, these hybrid models substantially improve the issue of unfaithful facts in baseline-generated conclusions.
When evaluating oncology facts, their correctness must be assessed by experts. Therefore, we show the conclusions generated by the system to experts for evaluation of clinical validity. We perform a human evaluation with two annotators (a radiologist and a final-year medical student) using an explanatory interface (Figure 1) to aid understanding. The assessment (Figure 2) considers oncologic correctness, non-oncologic correctness, and understandability with four rating levels: Without Findings (WE), Partial Response (PR), Stable Disease (SD), Progressive Disease (PD). A score of 0 is unacceptable, and 5 indicates complete correctness. Abstractive models score close to 5 for WE, while SD scores are comparable to human-written conclusions except for the baseline model. PR and PD summaries are more challenging due to complexity and limited training examples. Hybrid models outperform the baseline, with BERT2BERT+Ext+Ptr being the most accurate across patient degree categories.
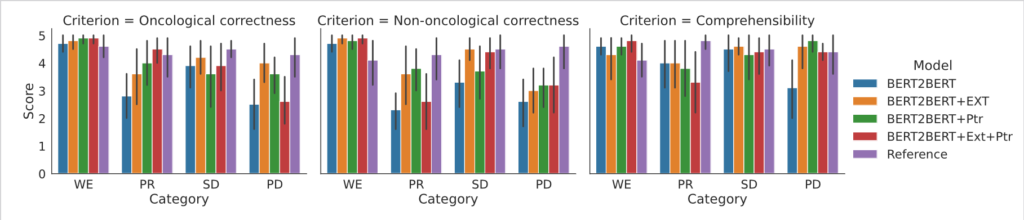
Figure 2: Average scores with standard deviation for the three criteria: Oncological correctness, non-oncological correctness and comprehensibility.
References
Liang, Siting, et al. “Fine-tuning BERT models for summarizing German radiology findings.” Proceedings of the 4th Clinical Natural Language Processing Workshop. 2022.
Weber, Tim Frederik, et al. “Improving radiologic communication in oncology: a single-centre experience with structured reporting for cancer patients.” Insights into Imaging 11 (2020): 1-11.