Gaze estimation error can severely hamper usability and performance of mobile gaze-based interfaces given that the error varies constantly for different interaction positions. In this work, we explore error-aware gaze-based interfaces that estimate and adapt to gaze estimation error on-the-fly. We implement a sample error-aware user interface for gaze-based selection and different error compensation methods: a naive approach that increases component size directly proportional to the absolute error, a recent model by Feit et al. that is based on the two-dimensional error distribution, and a novel predictive model that shifts gaze by a directional error estimate. We evaluate these models in a 12-participant user study and show that our predictive model significantly outperforms the others in terms of selection rate, particularly for small gaze targets. These results underline both the feasibility and potential of next generation error-aware gaze-based user interfaces.
We received the “Best Paper Award” at ETRA 2018 for this work. [read the paper]
We investigate different methods for adapting the interface and for compensating the gaze estimation error: (a) Naïve scales selection targets likewise for both dimensions and is evaluated in the user study with different error models; (b) the method Feit et al. [Feit et al. 2017] that scales targets based on the 2D error distribution extended for mobile settings; (c) our novel method PredictiveShift that shifts gaze based on a directional error estimate.
Our framework enables a new class of gaze-based interfaces that are aware of the gaze estimation error. Driven by real-time error estimation this approach has the potential to outperform state-of-the-art gaze selection methods in terms of selection performance with competing target sizes. We presented a first implementation of the framework and evaluated a naive target scaling approach with four methods to estimate the gaze error in a user study.
Elaborating on our findings, we developed and compared advanced error compensation methods. The results show both the real-time capability as well as the advantages of an error-aware interface, relying on gaze shifting and personalised training with a predictive model, in terms of selection performance and target sizes.
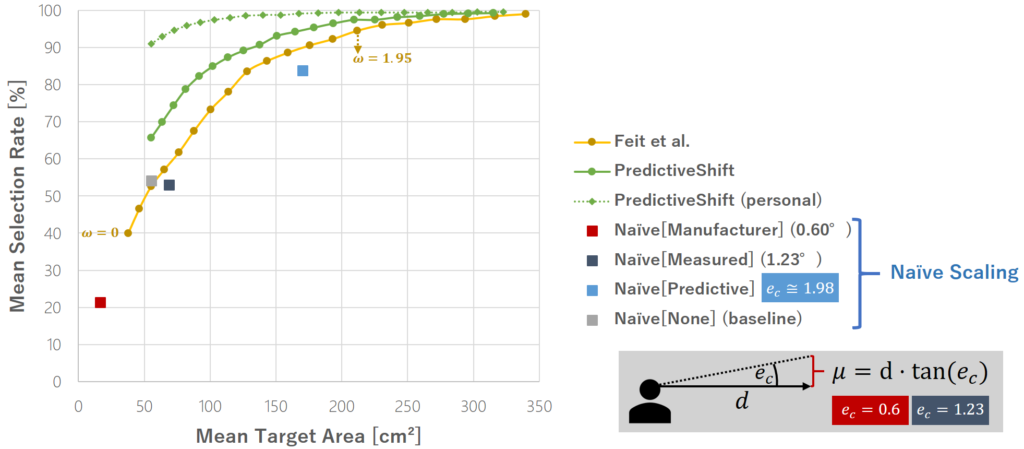
Mean selection rate off all methods in relation to the average target sizes. For the new compensation methods, we include the results for varying ω.
References
- Error-aware Gaze-based Interfaces for Robust Mobile Gaze Interaction. In: Proceedings of the 2018 ACM Symposium on Eye Tracking Research & Applications, pp. 24:1-24:10, ACM, 2018.
- Prediction of Gaze Estimation Error for Error-Aware Gaze-Based Interfaces. In: Proceedings of the Symposium on Eye Tracking Research and Applications, ACM, 2016.