Crowdsourcing is recently used to automate complex tasks when computational systems alone fail. In this project, we investigate how humans can effectively contribute to automate natural language translation. The envisioned goal is a hybrid machine translation service that incrementally adapts machine translation models to new domains by employing human computation to make machine translation more competitive. Therefore, we investigate efficient ways for domain adoption of neural machine translation systems using crowd-generated input data.
Poster at Collective Intelligence 2018
In this work, we investigated (1) whether a (paid) crowd, that is acquired from a multilingual website’s community, is capable of translating coherent content from English to their mother tongue; and (2) in which cases state-of-the-art machine translation models can compete with human translations for automation in order to reduce task completion times and costs.
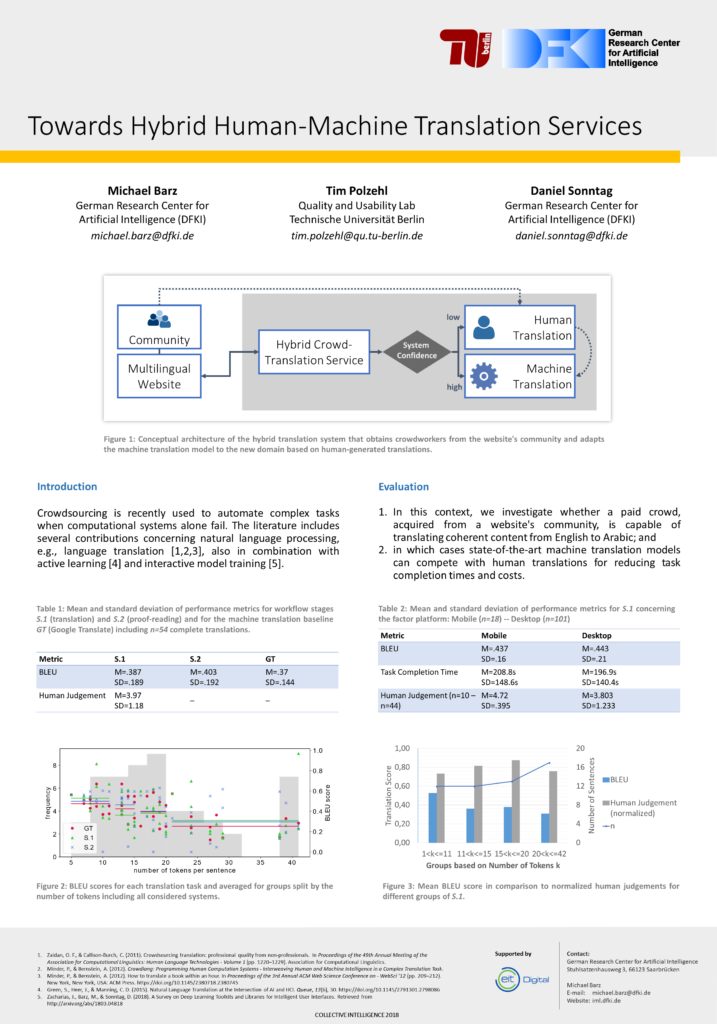
References
- Towards Hybrid Human-Machine Translation Services. EasyChair Preprint no. 333, 2018.
- Device-Type Influence in Crowd-based Natural Language Translation Tasks (short paper). In: Aroyo, Lora; Dumitrache, Anca; Paritosh, Praveen; Quinn, Alexander; Welty, Chris; Checco, Alessandro; Demartini, Gianluca; Gadiraju, Ujwal; Sarasua, Cristina (Ed.): Proceedings of the 1st Workshop on Subjectivity, Ambiguity and Disagreement in Crowdsourcing, and Short Paper Proceedings of the 1st Workshop on Disentangling the Relation Between Crowdsourcing and Bias Management (SAD 2018 and CrowdBias 2018), pp. 93-97, CEUR-WS.org, 2018.
- Incremental Domain Adaptation for Neural Machine Translation in Low-Resource Settings. In: Proceedings of the Fourth Arabic Natural Language Processing Workshop, pp. 1-10, Association for Computational Linguistics, 2019.
Contact
Michael Barz and Marimuthu Kalimuthu
Supported by
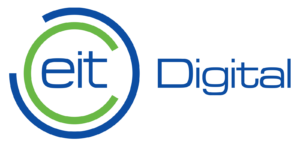
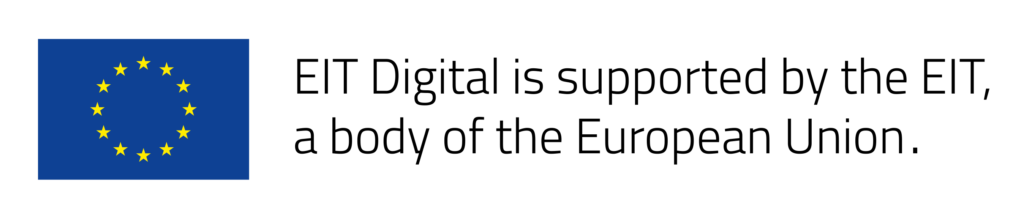