Funding Period: 10/2020 – 9/2023
To broaden the use of AI methods in medicine, networked, digital patient data is required, for example for use in disease diagnosis, personalized treatment, and faster drug discovery. Our project pAItient (AI innovation center with integrated, rights-protected environment for the development, testing, and clinical assessment of Al-based applications) establishes an innovation center for artificial intelligence at the University Hospital Heidelberg to provide a unified research infrastructure for the integration of AI solutions in healthcare. The goal is to automate the development of AI applications from the initial idea to their dissemination in everyday clinical practices.
Using transfer learning methods, i.e., the transfer of learned knowledge to another areas, researchers at DFKI are studying automated diagnostic techniques with detection based on clinical text and image data. The project also looks at ways to integrate other AI methods into the infrastructure. Testing and validation are ongoing in two areas: First, the extraction and summarization of medically relevant data from unstructured texts, and second, the analysis of ultrasound images of the carotid artery regarding the risk of a heart attack. The focus is on continuous improvement of the model performance by verifying the results through the involvement of users’ corrections. For more details on a clinical information extraction task see also this entry.
A sample use case
A class of machine learning models that generate new data based on patterns observed in a training dataset in the field of natural language processing (NLP) is called a generative model. In this context, one application of the generative model within the framework of the pAItient project aims to produce concise summaries of radiology reports. Various techniques are being investigated to improve the accuracy and comprehensibility of the generated summaries. Comprehensibility is checked by contrasting the generated summary with the original content of medical texts by highlighting the relevant input data, which also serves the purpose of verification. While the summaries generated by generative models achieve high static accuracy, in some cases, they are hard to follow or are not easily understood. This makes them unsuitable for the intended audience. A review by medical professionals is intended to address this issue. More details on the evaluation results by professionals can be found here.
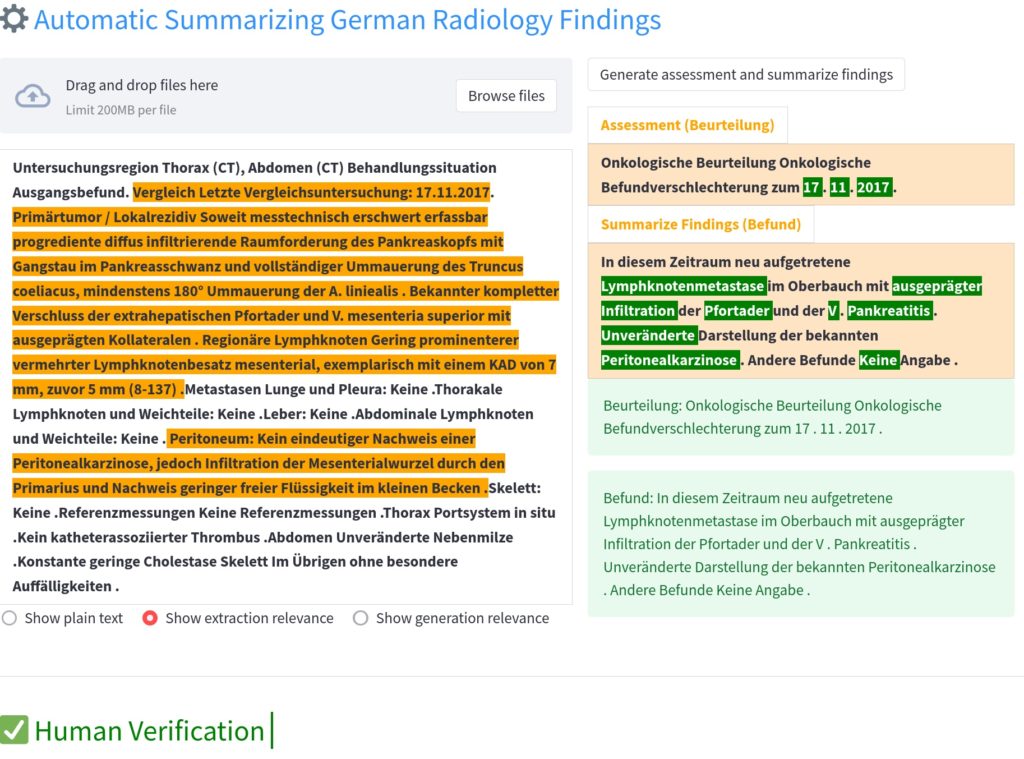
An example of an automated, comprehensible radiology report summary. During generation, the generative model extracts important segments (in green) from the original text (left) and inserts them into the summary (right)
References
Liang, Siting, et al. “Fine-tuning BERT models for summarizing German radiology findings.” Proceedings of the 4th Clinical Natural Language Processing Workshop. 2022.
Liang, Siting, Mareike Hartmann, and Daniel Sonntag. “Cross-domain German Medical Named Entity Recognition using a Pre-Trained Language Model and Unified Medical Semantic Types.” Proceedings of the 5th Clinical Natural Language Processing Workshop. 2023.
Weber, Tim Frederik, et al. “Improving radiologic communication in oncology: a single-centre experience with structured reporting for cancer patients.” Insights into Imaging 11 (2020): 1-11.
Richter-Pechanski, Phillip, et al. “A distributable German clinical corpus containing cardiovascular clinical routine doctor’s letters.” Scientific Data 10.1 (2023): 207.