AI Applications and use cases in sports at AAI from the University of OIdenburg and DFKI IML include monitoring and assisting the execution of the sport activities including crafting tailored training regimes, sport simulations, and strategic decisions in sport industry. In all applications and use cases, predictive (interactive) machine learning models are used in combination with multimodal multisensor interaction, typically in augmented reality (AR) or virtual reality environments. With our human-machine interaction and machine learning technology, we aim at supporting practical, real-life applications.
AI in Sports Activities
We believe that AI will revolutionise outdoor sports in particular, with innovative applications emerging in biking, climbing and other adventure activities. Our work is focused on AI for injury prevention, enhanced safety, better orientation, senso-motoric physical assistance, and health monitoring.
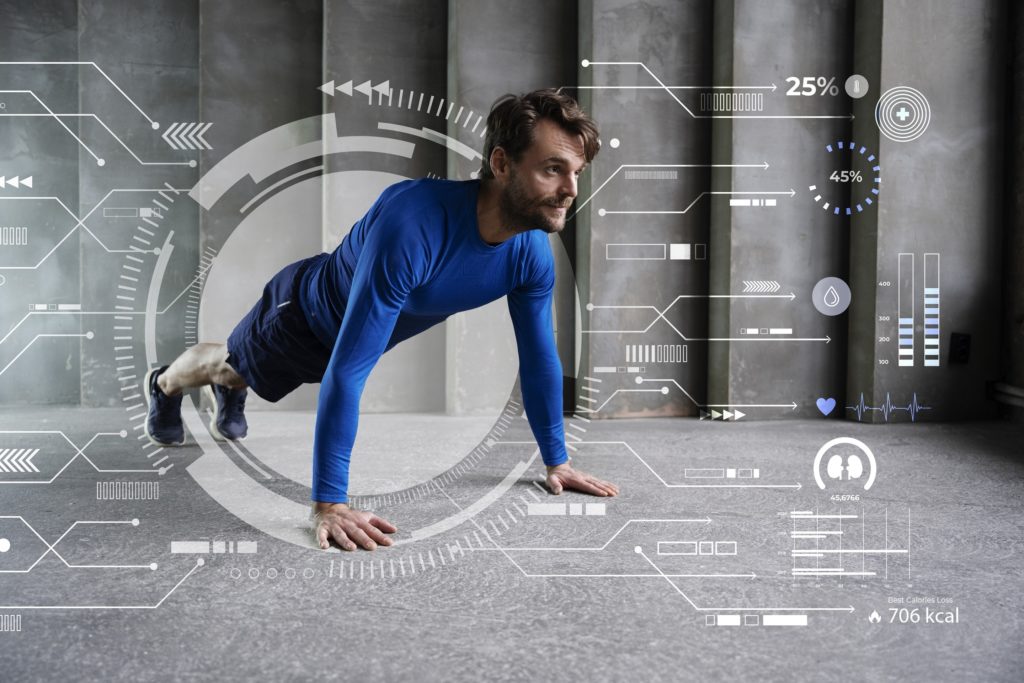
Biking:
AI-powered bicycles calculate the most efficient route to the user’s destination, promising a smoother and faster ride (backend application) and present the AI output in a multimodal intelligent user interface. Technologies: Eye Tracking, Head-Mounted displays.
In addition, AI-based suspension control supports safer rides (e.g., by automatically switching the fork and shock between open, pedal and lock modes, see this article).
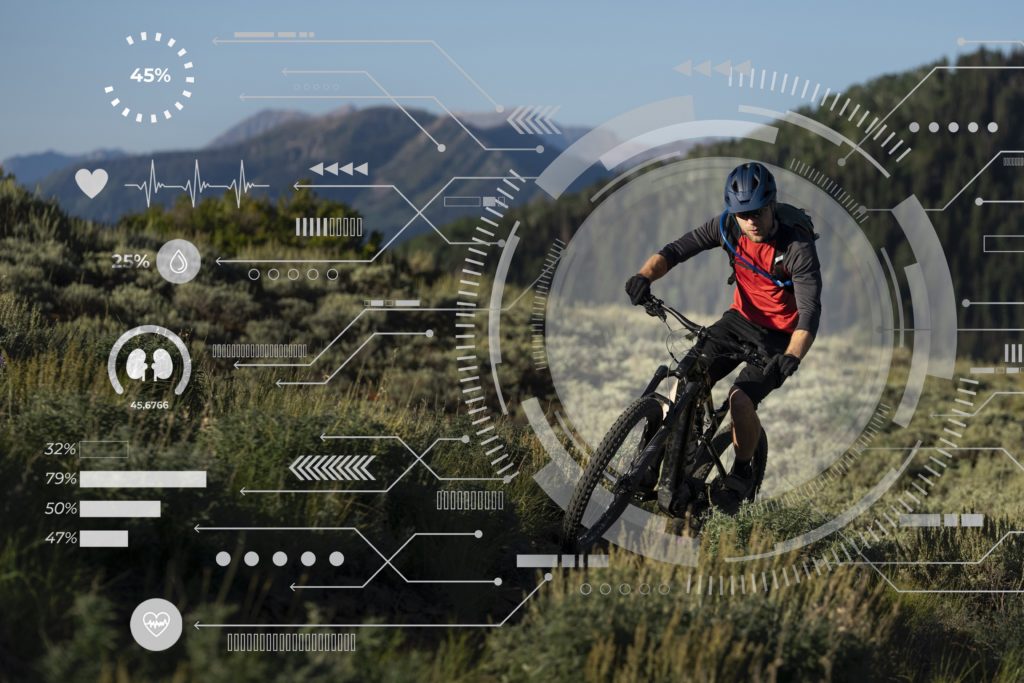
Rock Climbing:
Based on DFKI COS department’s survey of technology acceptance of climbers, we try to gain new insight in the acceptance of AI technology in climbing, when combined with IML technology.
Skiing:
Using mobile eye tracking and speech dialogue technology, our work focusses on AI for injury prevention, enhanced safety and better orientation while skiing.
AI in Sport Simulations:
AI can be used to create virtual reality environments for training and player development (improve player performance).
AI in Sports Industry:
AI can be used to analyse video material and other large amounts of data to identify patterns that can be used to make strategic decisions.
Relevant IML publications:
Sharon Oviatt, Björn Schuller, Philip R. Cohen, Daniel Sonntag, Gerasimos Potamianos, and Antonio Krüger (Eds.). 2017. The Handbook of Multimodal-Multisensor Interfaces: Foundations, User Modeling, and Common Modality Combinations – Volume 1. ACM Books, Vol. 14. Association for Computing Machinery and Morgan & Claypool. https://doi.org/10.1145/3015783
Hitesh Kotte, Florian Daiber, Milos Kravcik, and Nghia Duong-Trung. 2024. FitSight: Tracking and Feedback Engine for Personalized Fitness Training. In Proceedings of the 32nd ACM Conference on User Modeling, Adaptation and Personalization (UMAP ’24). Association for Computing Machinery, New York, NY, USA, 223–231. https://doi.org/10.1145/3627043.3659547
Michael Barz, Omair Shahzad Bhatti, Bengt Lüers, Alexander Prange, and Daniel Sonntag. 2021. Multisensor-Pipeline: A Lightweight, Flexible, and Extensible Framework for Building Multimodal-Multisensor Interfaces. In Companion Publication of the 2021 International Conference on Multimodal Interaction (ICMI ’21 Companion). Association for Computing Machinery, New York, NY, USA, 13–18. https://doi.org/10.1145/3461615.3485432
Sponsored by
2024-2027